One-stage Tumor Detection for Automated Breast Ultrasound Using Deep Convolutional Neural Network
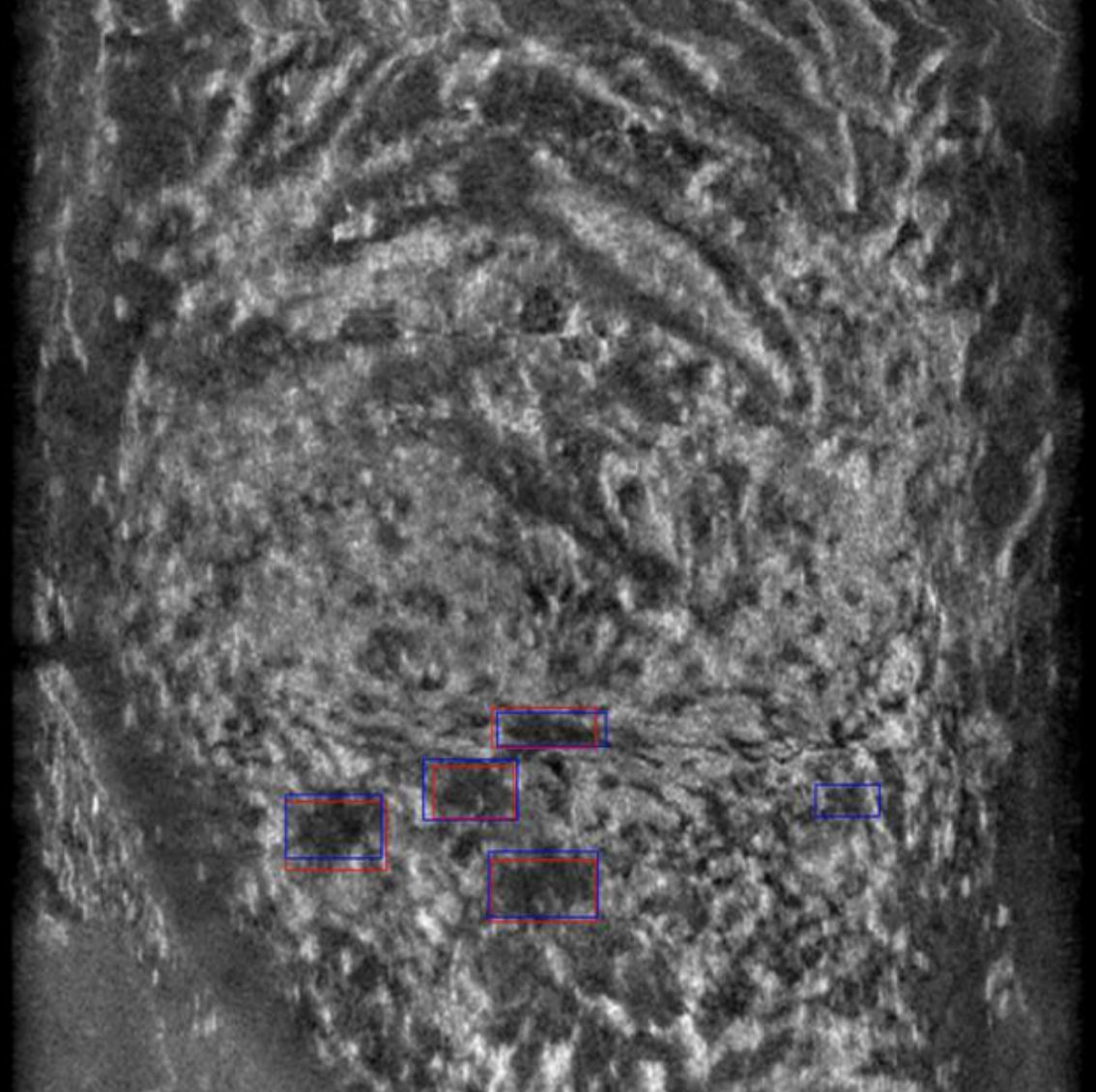
Software engineer with experience with Web Development, Internet Of Things and Machine Learning. Eagering to apply the learned to build practical tools for users. Fast learner with a can-do spirit and full of curiosity about new techniques.
The University of Texas at Austin
Master - Computer Science - -
National Taiwan University
Bachelor - Computer Science and Technology - -
Change Healthcare
Software Engineer- -
National Taiwan University
Research Assistant - -
Brogent Technology Inc.
Software Engineer - -
One-stage Tumor Detection for Automated Breast Ultrasound Using Deep Convolutional Neural Network
Computer-Aided Tumor Detection in Automated Breast Ultrasound using a 3-D Convolutional Neural Network
Tumor Diagnosis For 3-D Breast Elastography Image Using Convolutional Neural Network
WWOOF and Working Holiday
CSIE Student Association
One-stage Tumor Detection for Automated Breast Ultrasound Using Deep Convolutional Neural Network
Jan. 2019 - Aug. 2019
Abstract
The automated breast ultrasound (ABUS) had been widely used in breast examination. However, it is time-consuming for the physician to review hundreds of slices produced by ABUS. In recent, the computer-aided system based on the convolutional neural network (CNN) had been proven that it can assist effectively the physician for tumor detection and diagnosis on the medical image. Therefore, a computer-aided detection (CADe) system based on the one-stage 3-D convolutional neural network (CNN), 3-D You Only Look Once (3-D YOLO), is proposed in this study to locate suspicious lesions for the physician reviewing image. The particular characteristic of our system is that the whole ABUS is detected quickly in one take and the average detecting time of per ABUS image is 0.8 second. Moreover, the proposed system is also designed for smaller tumors to reduce the misdetection rate. In the proposed system, for achieving better performance, not only the one-stage YOLOv3 (You Only Look Once Version 3) object detection architecture is used and redesigned for smaller tumors, but also the essence of focal loss is applied to our loss function, and the scheme of cycle learning is designed for solving data imbalance problem. Before the tumor detection, the ABUS images are resized firstly to match the model shape. Then, the bounding boxes of tumor candidates are generated by the detection system. After that, the non-maximal suppression (NMS) is performed to eliminate the overlapping for determining the final tumor bounding box. Finally, our method achieves sensitivities of 98%, 95%, 90% with 3.8, 2.0, 1.0 false positives (FP) per pass, respectively. Compared to the previous works, the proposed CADe system is much better and faster.Computer-Aided Tumor Detection in Automated Breast Ultrasound using a 3-D Convolutional Neural Network
Sept. 2018 - Feb. 2019
Abstract
Automated breast ultrasound (ABUS) is a widely used screening modality for breast cancer detection and diagnosis. In this study, an effective and fast computer-aided detection (CADe) system based on the 3-D convolutional neural network (CNN) is proposed as the second reader for the physician to downgrade the reviewing time and misdetection rate. Methods Our CADe system consists of the sliding window method, a CNN- based determining model, and a candidate aggregation algorithm. First, the sliding window is performed to split ABUS volume into volumes of interest (VOIs). Afterward, the VOIs are selected as tumor candidates by our determining model. For achieving better performance, the focal loss and ensemble learning is used to solve data imbalance and reduce false positive (FP) and false negative (FN). Because there are several candidates part of same tumor selected, and they may overlap each other, a candidate aggregation method is applied to merge the overlapping candidates into the final detection result. Results In experiments, 165 and 81 cases are utilized for training and evaluating system performance. On evaluation with 81 cases, our system can achieve sensitivities of 100% (81/81), 95.3% (77/81), and 90.9% (74/81) with FPs per pass (per case) 21.6 (126.2, 6.0 (34.8), and 4.6 (27.1) respectively. According to the results, the number of FPs per pass (per case) can be diminished by 56.8% (57.1%) at the sensitivity of 95.3% based on our tumor detection model. Conclusions In conclusion, the proposed CADe system using 3-D CNN with the focal loss and ensemble learning has the capability of achieving better performance than previous works.Tumor Diagnosis For 3-D Breast Elastography Image Using Convolutional Neural Network
Apr. 2018 - Oct. 2018
Abstract
Recently, the breast elastography techniques had become a helpful examination for cancer diagnosis. In this study, we proposed a CAD diagnosis system based on the 3D convolution neural network to classify a tumor into benign or malignant using the B- mode images and elastographic images. At first, the U- Net segmentation method is used to extract the tumor region from 3-D B-mode images. After generating the tumor region, the mask images contain complete shape features. Thus, the tumors are diagnosed in the proposed CAD systems using B-mode images (B), elastographic images (E), and segmented B-mode tumor images (T). In addition, the ensemble method is adopted to reduce prediction variance and enhance diagnosis performance. In this experiment, a totally 83 biopsy proved lesions composed of 63 benign tumors and 20 malignant tumors are used to evaluate our proposed method. According to results, the best diagnosis performance is using the ensemble method combined with B-mode images (B), elastographic images (E), and segmented B-mode tumor images (T) and the accuracy, sensitivity, specificity, and AUC are 87.95% (73/83), 95.00% (19/20), 85.71% (54/63), and 0.9464, respectively.NTUCOOL
May 2018 - Jan. 2019
4-D Rides: Tim’s Rescue & Arctic 1
Jan. 2014 - Mar. 2016
Central Control Systems Programming
Tim’s Rescue is a 4-D ride in Yumble, Roermond, Netherlands.
Arctic 1 is a 4-D ride in Wildlands Adventure Zoo Emmen, Emmen, Netherlands.
▲Tim’s Rescue design
▲Video: Tim’s Rescue
▲Yumble, Roermond
▲Video: Arctic 1
▲Inside of Arctic 1
▲Outside of Arctic 1 in Zoo Emmen
Water Distribution
July 2012 - Jan. 2013
Digital Face Makeup (Digital Image Processing)
Spring 2013
Head Bounce (Computer Graphic)
Fall 2012
BEWSBB (Computer Network Lab)
Spring 2012
MUSOU (Game Programming)
Fall 2011
Attack Danbo (Digital Visual Effects)
Fall 2012
WWOOF (Volunteering) and Working Holiday
2016 - 2018
Volunteered and worked in various industries, such as ranching, farming, and dining, communicated with travelers and local residents in Japanese and English, with full exposure to foreign cultures
▲3 Days Camping, Uluru, Australia
▲Glacier Hiking, Franz Josef Glacier, New Zealand
▲Ranching Family, Kanazawa, Japan
CSIE Student Association
2009 - 2013
Public Relations Manager
CSIE Student Association, 2011 - 2012
Chief Convener
CSIE Exhibition in NTU Azalea Festival, 2012
Artistic Designer
Week of CSIE, 2011
Instructor and Artistic Designer
Summer Camp of CSIE, 2010, 2011, and 2012
Basketball Team Manager
Girl Basketball Team, Dept. of CSIE , 2011 - 2013
Dance, Band, and Musical Performer
▲Instructor, CSIE Camp
▲CSIE booth in NTU Azalea Festival
▲Band, Night of CSIE
▲Dancer, Night of CSIE
▲Cactuses for sale, Week of CSIE
▲Marketing Material Design, Week of CSIE
▲Marketing Material Design, CSIE Camp
▲Marketing Material Design, NTU Azalea Festival